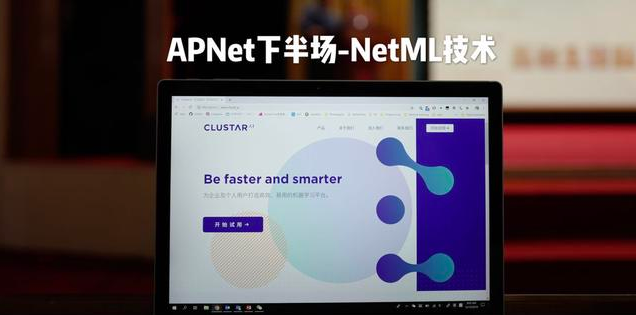
On December 15, 2018, APNet China study BBS Forum China network in Beijing xiangshan convention center, the conference was hosted by APNet, CCF, the Internet branch of CCF, the network and data communications branch of ACM SIGCOMM China, BBS invited bytes to beat, alibaba, baidu, huawei, China mobile, the Mellanox big-name industry such as Daniel, as well as the Hong Kong university of science and technology, tsinghua university, national university of defense technology experts and scholars, such as around the RDMA and NetML two themes brought a audio-visual feast for you, nebula Clustar as RDMA technology and NetML technology explorer, also involved in this event,
This article for the second half of the dry NetML technology sharing, in the first half of the dry RDMA technology in another article presented alone, didn't see a friend can directly to land nebula Clustar public official number: "Clustar2018" or zhihu institutions, consult,
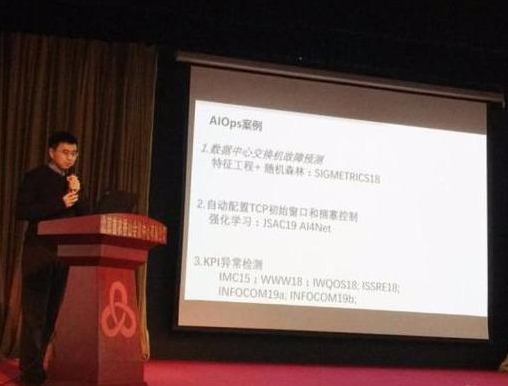
About NetML technical meeting minutes in the second half:
PeiDan professors from tsinghua university to share the theme of "Autonomous IT Operations through Machine Learning" excellent speech, speech is mainly around the unmanned Operations area, professor PeiDan that Ops Ops is one of the core technology of digital economy, along with the application of more and more complex, more and more large and complex network system, distributed systems across the protocol layer is more and more popular, and many other factors, makes the operational accidents frequently occur, so you need a lot of manpower, material resources to solve the problem of fault, at the same time also very difficult to find fault, lead to the result of an important reason is the different operational system exists in the monitoring indicators, abnormal, one thousand different log on different systems and devices, and so on and so forth, with the mature of AI technology, operational technology development trend is from human flesh Operations to Ops, AI AI will gradually replace the human, IT can quickly solve fault, finally realizes the unmanned Operations, but the AI is only good at solving some of the problems in the field of Operations, different scenarios require different Machine algorithm, to this, the professor PeiDan through three AI Ops case to illustrate, switch failure prediction from the data center, automatic configuration of TCP congestion control and intelligent abnormal detection algorithm, AI practical application in the field of Operations, in the first case, through the characteristics of the engineering and random forest algorithm switches fault detection scheme, obtain very good effect; The second case, professor PeiDan through training to put forward a dynamic adjust the TCP window style, the very good solution to the traditional TCP window is fixed in advance in the network server problems, although, now the congestion control algorithm has been optimized, but also can't universality, professor PeiDan by reinforcement learning method is put forward, the configuration flow startup and congestion control to adapt to specific application scenario, the application case in the baidu search, the optimized algorithm will increase the performance of TCP communications by 29%, this in the field of traditional TCP communications is a very great achievement, the last case, professor PeiDan raised a set of intelligent algorithm, it has no need for any configuration and annotation, from simple method for anomaly detection to supervise the anomaly detection, unsupervised anomaly detection, assisted with feedback, to anomaly detection of millions of curve, finally realizes the automatic adaptation curve changes, through the automatic selection algorithm and parameter combination, multi detector as features combined with machine learning, avoid using artificial participation, so as to decrease the difficulty of operations for a complex data distribution, through the VAE model can be mapped to a more precise distribution, improve the accuracy of detection, nebula Clustar thinks, the applications of AI techniques in the field of operations in the future an important development direction, can greatly improve enterprise operational maintenance efficiency and production capacity, reduce operational costs,
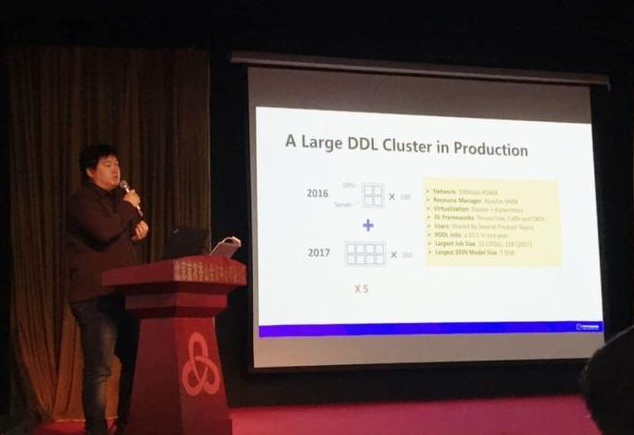
阿里巴巴高级技术专家刘洪强博士为大家分享的是"Tiresias: A GPU Cluster Manager for Distributed Deep Learning"的主题演讲,刘洪强博士提出深度学习训练集群中的一个主要目标就是尽可能减少每个深度学习任务的排队时间,而针对深度学习的调度方案则是实现这一目标的一个有效方法,现阶段,深度学习任务往往同时依赖RDMA以及容器等基础技术,如何使得这两个技术可以平滑地结合在一起,从而使整个集群的任务具有客观理性,调度性成为当前的一个难题,为了解决这一问题,刘洪强博士首先提出了FreeFlow,FreeFlow通过FreeFlowRouter(FFR)这一层,拦截容器中ibverbs的接口调用,并在FFR中做出相应的操作,本质上FreeFlow使得容器内对queue pair的操作被代理到FFR中的queue pair上,并且将容器内应用的内存映射至FFR,从而提升原生的RDMA性能,FreeFlow 保证了容器的可控性,隔离性以及可迁移性,星云Clustar认为,RDMA与容器技术相结合是未来的一个趋势,我们在这一方面也有着自己的思考,之后,刘洪强博士讲述了他的第二个工作:Tiresias,深度学习的任务执行时间往往是不可预测的,比如有些任务经常是机器学习科研工作者尝试model性能的任务很快就会被主动kill掉,Tiresias不依赖于任务的执行时间,它通过时间和空间这两个维度的优化算法来优化任务的调度,从而减少任务的等待时间,
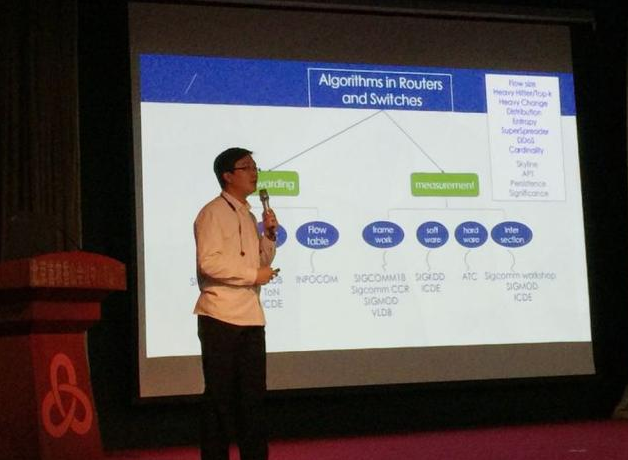
最后一位分享嘉宾是来自北京大学的杨仝教授,他分享的题目是"Empowering Sketches with Machine Learning for Network Measurements",其内容为通过Sketch数据结构来进行网络测量,网络测量任务往往需要识别流的大小,区分大小流,同时也需要知道流的个数,现有的方案都基于数据包采样,没有办法得到很好的测量精度,现在,大家普遍认为Sketch是一个比较好的方法,Sketch是一种类似Bloom Filter的数据结构,体积小效率高,已经有大量实际部署的应用使用Sketch来解决不同的网络测量任务,比如heavy changes sketch用于检测流大小变化非常剧烈的流,从而定位可疑流量,杨仝教授接着介绍了一个通用框架(framework),将机器学习的方法来用于Sketch,首先通过采样,然后根据采样到的数据集来进行学习,并且构建Learning Sketch,不同的Sketch需要选择不同的feature,然后进行训练,产生最终的Sketch,杨仝教授强调当传统生成的Sketch不准的时候,这种基于机器学习的方法可以发挥很大的功效,提升Sketch的精确度,杨仝教授也介绍了Sketch几种常见的使用场景,比如估测Top-k的流量,数流的个数,以及cardinality统计,同时,也分享了在实际流量下的实验室数据,星云Clustar认为,网络测量对于理解网络,从而更好的运维,优化网络具有非常重要的意义,Sketch作为一种大规模网络测量的有效手段,极大提高了网络测量的可行性,
CodePudding user response:

CodePudding user response:
