I need to calculate a huge table value (157954 rows and 365 columns) by checking three conditions in a dataframe with 11 mil rows. Do you have any way to speed up the calculation, which is taking more than 10 hours now?
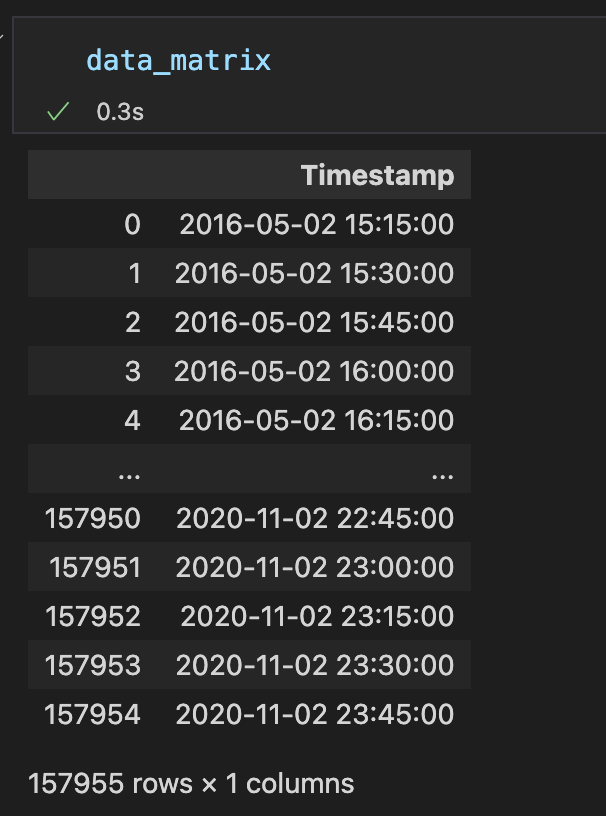
I have 367 stations in total.
for station in stations:
no_pickup_array = []
for time_point in data_matrix['Timestamp']:
time_point_2 = time_point timedelta(minutes=15)
no_pickup = len(dataframe[(time_point <= dataframe["departure"]) & (dataframe["departure"] < time_point_2)
& (dataframe['departure_name'] == station)])
no_pickup_array.append(no_pickup)
print(f"Station name: {station}")
data_matrix[station] = no_pickup_array
I appreciate any of your help.
CodePudding user response:
The main problem is the right-hand-side of the no_pickup
assignment expression which is algorithmically inefficient because it makes a linear search while a logarithmic search is possible.
The first thing to do is to do a groupby
of dataframe
so to build an index enabling to fetch the dataframe subset having a given name. Then, you can sort each dataframe subset by departure so to be able to perform a binary search enabling you to know the number of item fitting the condition.
The index can be built with something like:
index = {name: df for name, df.sort_values('departure')['departure'].to_numpy() in dataframe.groupby('departure_name')}
Finally, you can do the binary search with two np.searchsorted
on index[station]
: one to know the starting index and one to know the ending index. You can get the length with a simple subtraction of the two.
Note that you may need some tweak since I am not sure the above code will works on your dataset but it is hard to know without an example of code generating the inputs.
CodePudding user response:
You're indexing the dataframe list with a boolean (which will be zero or one, so you're only ever going to get the length of the first or second element) instead of a number. It's going to get evaluated like so:
len(dataframe[(time_point <= dataframe["departure"]) & (dataframe["departure"] < time_point_2) & (dataframe['departure_name'] == station)])
len(dataframe[True & False & True]) # let's just say the variables work out like this
len(dataframe[False])
len(dataframe[0])
This probably isn't the behavior you're after. (let me know what you're trying to do in a comment and I'll try to help out more.)
In terms of code speed specifically, &
is bitwise "AND", in python the boolean operators are written out as and
, or
, and not
. Using and
here would speed up your code, since python only evaluates parts of boolean expressions where they're needed, e.g.
from time import sleep
def slow_function():
sleep(3)
return False
# This line doesn't take 3 seconds to run as you may expect.
# Python sees "False and" and is smart enough to realize that whatever comes after is irrelevant.
# No matter what comes after "False and", it's never going to make the first half True.
# So, python doesn't bother evaluating it, and saves 3 seconds in the process.
False and slow_function()
# Some more examples that show python doesn't evaluate the right half unless it needs to
False and print("hi")
False and asdfasdfasdf
False and 42/0
# The same does not happen here. These are bitwise operators, expected to be applied to numbers.
# While it does produce the correct result for boolean inputs, it's going to be slower,
# since it can't rely on the same optimization.
False & slow_function()
# Of course, both of these still take 3 seconds, since the right half has to be evaluated either way.
True and slow_function()
True & slow_function()