title: electromyographic signal prosthesis controller design based on STM32
Key words: ARM; ; multi-channel semg Feature extraction; The prosthetic control; The BP neural network
This topic put forward a kind of myoelectricity prosthetic controller design scheme based on ARM, with only two electrical sensor to realize the on-line identification of five kinds of action mode, are respectively on the palm of your hand, then, hand clenched, his palm and no action, the system is divided into hardware part and software part and hardware part mainly includes: system power supply module and microprocessor minimum system module, signal amplification adjustment module and serial communication module, etc.; Software part mainly includes: A/D signal collection, IIR filter, signal feature extraction and BP neural network recognition, etc.,
System first by two electrical sensors sampling called lateral side wrist flexor wrist flexor and ruler of SEMG signal, because the raw SEMG signals is weak and the positive and negative, so want to signal amplification and adjustment, and then input to the A/D sampling ARM kernel STM32 processor, and then, in the processor, IIR digital filter was used to collected SEMG signal band-pass filter, and extract the characteristic values of four kinds of time domain and frequency domain signal amplitude absolute mean value (MAV) respectively, and the standard deviation (SD) signal, the median frequency (MF) and mean power frequency (MPF), then, will be 2 SEMG signals of the four features of A 8 D vector, using Matlab training BP neural network weights and threshold coefficient, according to BP network input layer to hidden layer, hidden layer to output layer transfer function formula of STM32 weights and threshold values for the corresponding operation, corresponding to the on-line identification of five kinds of action results, the result according to the serial port output control signal control prosthetic limbs make corresponding action,
The emg sensor:
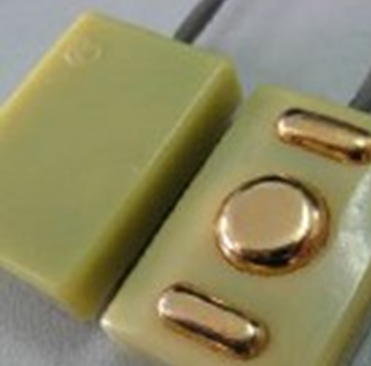
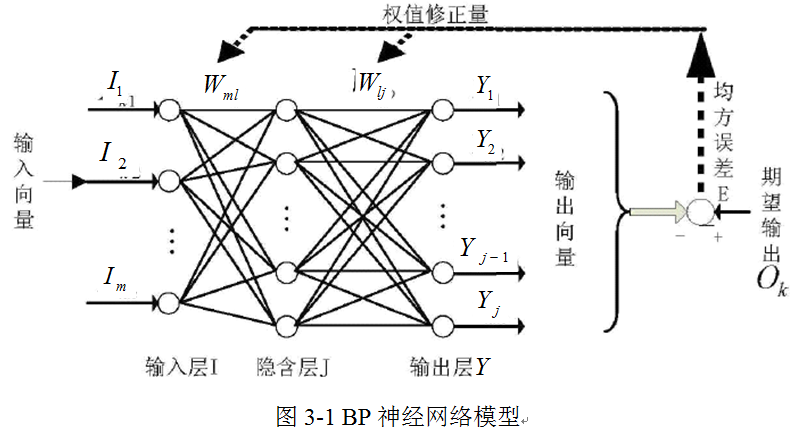
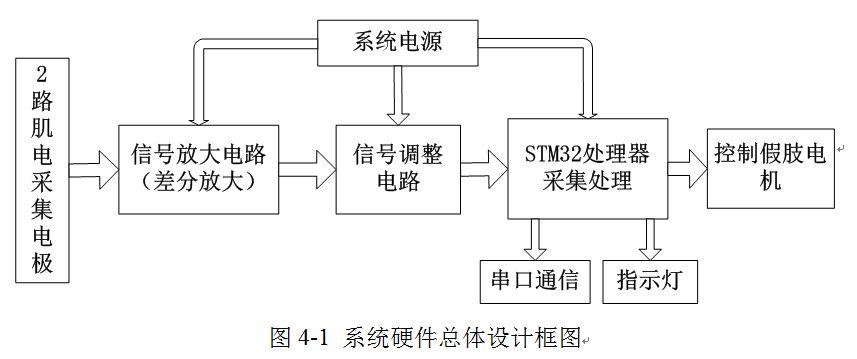
The overall hardware design:
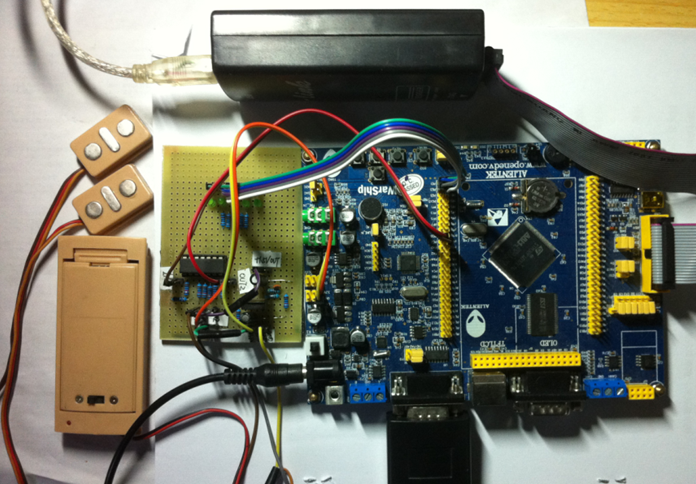
Online experiment, the on-line identification, and the LED indicator, and drive the rubber hand action:
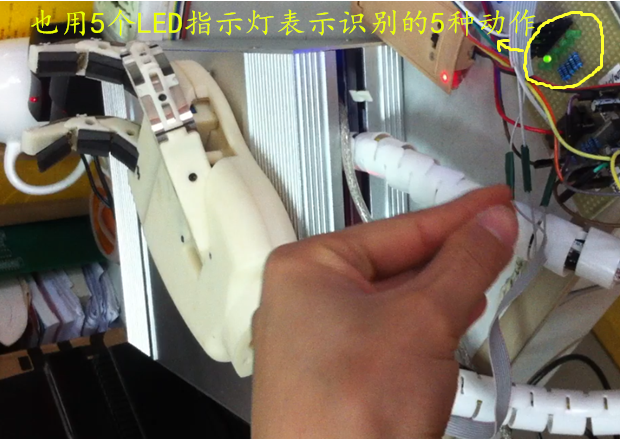
CodePudding user response:
Hello, I'm going to graduation design do a similar electromyography collection control system, don't know if the original poster have some resources


CodePudding user response:

CodePudding user response:
Senior, to meet you here, say hello
CodePudding user response:
Senior. We ask you the question when you have time, I do you don't know why light is not on this project? Ask elderCodePudding user response:
With the arduino how ahCodePudding user response:
Mark, have specific needs to contact you again later