The import numpy as np
Import the random
Def func1 (z) :
Total=0
For I in range (0, z.s considering) :
[I] total=z * * 2 + total
Return the total
The class PSO:
Def __init__ (self, the size, dim, iter_num, C1 and C2, W, X_max, X_min, V_max, V_min) :
Self. Size=size
Self. Dim=dim
Self. Iter_num=iter_num
The self. The C1=C1
The self, C2=C2
Self. W=W
Self. X_max=X_max
Self. X_min=X_min
Self. V_max=V_max
Self. V_min=V_min
Self. X=np. Random. The random ((self. The size, the self. Dim)) * (X_max - X_min) + X_min
Self. V=np. Random. The random ((self. The size, the self. Dim)) * (V_max - V_min) + V_min
Self. P=self. X
Self. P_best=[func1 (self. X [I]) for I in range (0, the self. The size)]
Self. G_best=self. P_best [0] + 1
For I in range (0, the self. The size) :
If self. P_best [I]Self. G=self. [I] p
Self. G_best=self. P_best [I]
Self. Gb=[]
The self. The fitness=0
Def update (self) :
For I in range (self. Iter_num) :
For j in range (self. The size) :
The self. The fitness=func1 (self. X [j])
# update individual optimal location, optimal value
If the self. The fitness & lt; Self. P_best [j] :
Self. P=self [j]. [j] x
Self. P_best [j]=self. Fitness
# update group, the optimal location, optimal value
If self. P_best [j]Self. G=self. P [j]
Self. G_best=self. P_best [j]
# update speed, position
Self. V=self [j]. W * self in v + self [j]. The C1 * random in the random () * (self. P [j] - self. X [j]) \
+ self. C2 * random. The random () * (self. G - self. X [j])
Self. X [j]=self. V + self [j]. X [j]
# boundary conditionsFor k in range (self. Dim) :
If self. V [j] [k] & gt; Self. V_max or self. V [j] [k]Self. V [j] [k]=random. The random () * (self. V_max - self. V_min) + self. V_min
If self. X [j] [k] & gt; Self. X_max or self. X [j] [k]Self. X [j] [k]=random. The random () * (self. X_max - self. X_min) + self. X_min
Self. Gb. Append (self g_best)
Return the self. J g, self. G_best
The from PSO import PSO
Size=10
Dim=3
Iter_num=200
C1=1.5
C2=1.5
W=0.8
X_max=20
X_min=- 20
V_max=10
V_min=- 10
Pso=pso (size, dim, iter_num, C1 and C2, W, X_max, X_min, V_max, V_min)
Best_pos, best_fitness=pso. The update ()
In addition, I found a problem, why this way only changed the value of the a and b values has changed?
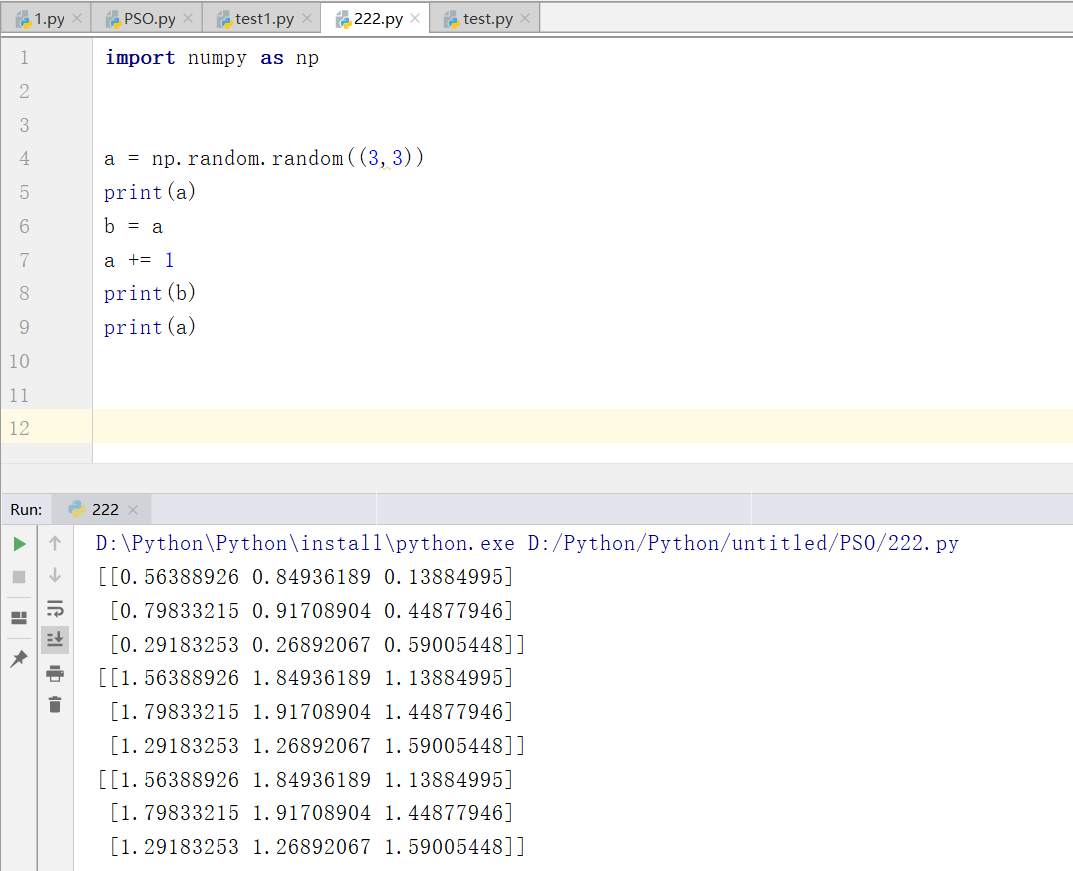
And this kind of situation, both values are not binding
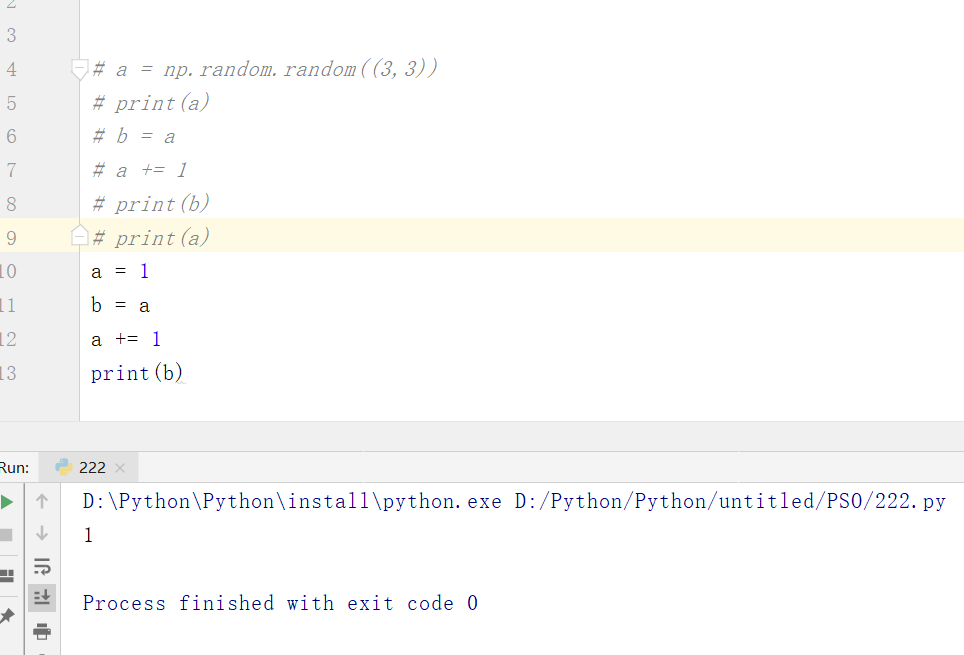
Does anyone know?